News
How to Leverage Data Analytics in Your Food Delivery App
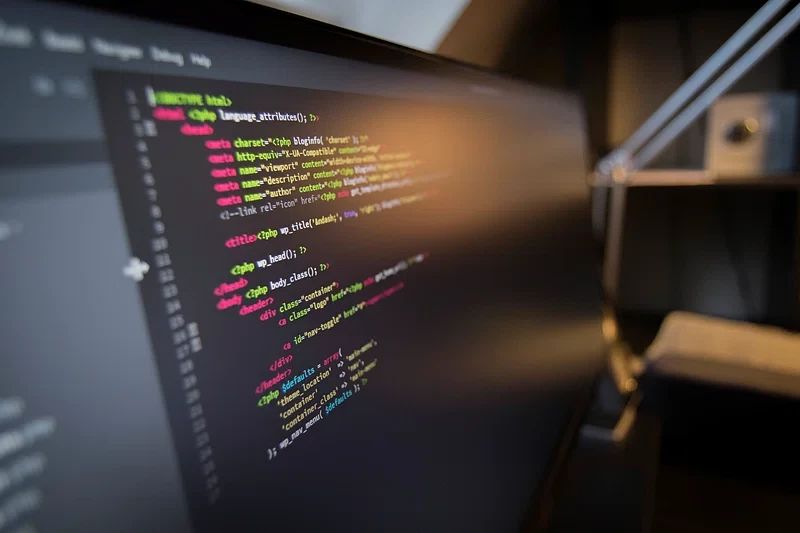
Introduction to Data Analytics in Food Delivery Apps
In today’s fast-paced digital landscape, food delivery apps are transforming how we enjoy our favorite meals. At the heart of this revolution lies data analytics — a powerful tool that enables businesses to better understand their customers, streamline operations, and enhance user experiences. By harnessing vast amounts of data generated through user interactions, food delivery apps can gain invaluable insights into customer preferences, order patterns, and market trends. For instance, utilizing services from https://celadonsoft.com/solutions/food-delivery-app-development-services can help in implementing advanced analytics features that drive customer satisfaction and loyalty.
Data analytics allow finding those key performance indicators necessary for success, such as the most ordered dishes, the most active time to place orders, and who one’s customers are. Such information ensures that the app developers optimize their service with the intention of ensuring that users get personalized recommendations or have their food delivered on time. Moreover, predictive analytics is possible to estimate demand, allowing a restaurant or delivery firm to manage its inventory and decrease food waste.
The heat of competition is gradually increasing in the food delivery industry, making the use of data analytics not a luxury but an imperative. Embracing this technology will make companies keep up with loyalty, satisfaction, and growth in the highly confronted market. Further, we will discuss some practical strategies for integrating data analytics into your food delivery app to maximize its potential.
Data Analytics: Importance for Food Delivery Services
Data analytics has emerged as a game-changer in food delivery services, moving at a speed that has never been seen before in the world. To put it in simpler words, data analytics helps transform raw data into actionable strategies which have the amazing potential to improve operational efficiency and customer satisfaction on the bottom line. Businesses can offer exactly what their customers want by deciphering consumer behavior, identifying delivery patterns, and peak ordering times.
For instance, data analytics help food delivery apps understand what type of dishes or cuisines are liked in which demographics, and therefore, where marketing campaigns should be run. Additionally, it aids in route optimization during delivery to reduce wait times and enhances the reliability of the service level, surely essential factors in customer retention in a competitive environment.
Analytics in real time can also help companies measure key performance indicators and customer feedback, which help the company make rapid adaptation and improvements. Knowing trends and seasonality also helps in inventory control so that products in high demand will be available while minimizing wastage of products. In conclusion, data analytics levers in the food delivery industry prove to enhance profitability and drive long-term growth due to improved customer experiences.
Key Metrics to Track in Food Delivery Apps
- Order Conversion Rate: The percentage of users who have added items to their cart and completed their purchase. A low rate might indicate an issue with your checkout process or the usability of your app.
- Customer Retention Rate: This metric shows how many customers continue to reuse your app over time. Strong retention indicates very satisfied customers who possess brand loyalty; poor retention may suggest room for improving the experience.
- AOV: The average order value. Obviously, the more the AOV increases, the greater the revenue will be because of exponential growth; develop upselling and cross-selling methods to increase this number.
- Delivery Time: Time from ordering to delivery. Food businesses relay on getting their food out hot. Faster delivery allows for a better customer experience.
- CSAT: Customer feedback data provides insights into satisfaction. This score can be used to identify areas that require improvement.
Types of Data to Collect from Users
Thus, the identification and collection of various types of data about users will be of paramount importance for leveraging data analytics in an effective manner in your food delivery app and boosting customer experience and business growth.
Demographic data can first be collected: age, gender, and location. This shall enable more focused marketing and also create menu items to meet the needs of such groups of customers.
Record user interaction with the application. Monitor patterns such as ordering history, browsing, and time spent on different sections of the application. Such information points to the favorite dishes ordered, the highest ordering hours, and helps identify the bottle-necks in customer’s journey.
It would also be useful to collect ratings and reviews for feedback. Understanding satisfaction and areas that need improvement is crucial for retaining customers and expanding the customer base. And last but not least, there is transaction data: payment methods, average order values, etc. Analytics based on this might surface trends, thus giving meaning to pricing strategies and promotions.
Finally, consider adding in social media data to assess customer sentiment and engagement. If you combine the above types of data, your app can create a personalized experience for users that meets their needs but at the same time heightens their loyalty and increases revenue.
Leverage Customer Feedback to Drive Data-Informed Decisions
Success in food delivery is all about understanding customer sentiment. Raw data could be transformed into actionable insights by using customer feedback for the development of your app and enhancing the user experience. First of all, provide the feedback feature right inside your app, where a simple star rating, an elaborate review, or even a quick survey provided at the end of every order has great importance.
After gathering this feedback, analytics tools sort out and categorize all the responses. Generally identify trends and themes; pain points on delivery times or dishes doing well might be some commonalities surfacing from this qualitative data that will help in fixing routes for delivery or improving restaurant menus.
Also, you can integrate the algorithms of sentiment analysis that quantify the emotions of customers behind the feedback. This would help to pinpoint not only the points of satisfaction but also bring to view some submerged issues which may not be visible.
With continuous monitoring and response to customer feedback, you create a dynamic feedback loop that engenders loyalty. Customers love to have their voices heard, assuring you of better retention and more referrals. In the cacophony of competition, only those who can listen intently and act swiftly will rise above the din.
Improve User Experience with Personalization
In the fierce competition characterizing the space of food delivery apps, personalization turned out to be one of those critical differentiators, while data analytics is the wind beneath its wings in the art of crafting bespoke user experiences. Using data from customer order history to preferences, even time of day, the apps curate personalized menus that would strike a chord with individual tastes. For instance, if a user frequently orders vegan food, the application should be able to suggest other items related to that particular dish or even recommend new places offering vegan cuisine, thereby giving him a sense of comfort and anticipation.
Predictive analytics will also forecast cravings depending on user behavior. If a customer orders pizza every Friday evening, for instance, the app should pop up notifications or special offers right on cue as the weekend is just about to start, subtly prompting them to make a purchase.
Personalization could also be extended by the use of machine learning algorithms to learn from interactions continuously; hence, recommendations change over time. Segmentation of users into quite a few distinct categories includes health-conscious eaters, adventurous foodies, and budget-conscious diners-allows each user to feel special and looked after. Loyalty and engagement would follow. Eventually, the customer feels understood and is well taken care of; he would surely come back for more-the casual diner turning into a patron.
Data-Driven Delivery Operations Optimization
- Route Optimization: Utilize current traffic conditions and patterns of past deliveries to determine the most efficient routes. These will, in turn, cut down delivery times and decrease fuel costs.
- Demand Forecasting: Study orders for trends and seasonality to anticipate peak periods, which would call for staffing and inventories accordingly.
- KPIs: Keep track of metrics regarding delivery time, order accuracy, and customer feedback as a way to understand where one fails.
- Customer Segmentation: Segment the customers based on their preferences and behavior with the help of data to enable personalized marketing and service offers.
- Driver Performance Analyses: Delivery personnel are tracked through insights from data with a view to enforcing accountability and designing better training programs to bring in upgraded service quality.
Case Studies: Successful Implementation of Data Analytics
Food ordering and delivery applications have become an extremely competitive space, where a few firms have learned to leverage data analytics for continuous improvement and growth. Take DoorDash, for example. The site used customer data to enhance its delivery routes. In fact, it managed to reduce times by an astonishing 30%. A predictive analytics model predicts peak times and customer preferences, and popular items are then stocked and ready for order, boosting volume.
Another successful example is the use of machine learning algorithms that analyze customer behavior by Uber Eats for the optimization of menu recommendations. By analyzing previous orders, how users interact with the app, or even social media trends, the application provides personalized suggestions that result in increased satisfaction of the users and higher average order sizes.
While Grubhub does the same thing by leveraging data analytics, it understands community preferences and identifies local restaurants to create mutual wins for both consumers and businesses. Such strategic action would heighten customer loyalty and add to the market reach of Grubhub.
These two case studies amply demonstrate how pragmatic implementation of data analytics leads to enhanced operational efficiency, higher levels of customer engagement, and finally, profitability in the food delivery industry.
Challenges in Leveraging Data Analytics
While food delivery apps can be truly game-changing, there are a considerable amount of challenges in using data analytics. Among the most smacking is the issue of the quality of data: a single piece of incorrect or missing information might corrupt the outcome and result in misinformed decisions. A certain example is that if user preferences are logged in error, menu offerings and customer satisfaction would go for a toss.
Another challenge might be integrating these sources of data. Generally, a food delivery application would generate its data from customer feedback, order history, social media conversations, and so on. Ensuring that these diverse sources talk well to each other for a holistic view is bound to be complex and resource-heavy.
Moreover, the fast nature of food delivery tends to batter analytics systems. To understand changing consumer trends, real-time data is required, and this is quite an expensive and laborious task to maintain the infrastructure.
Finally, there is the huge concern of data privacy. The increasing regulations like GDPR raise challenges for food delivery apps to find that fine line between leveraging data for customer experiences and respecting user privacy. This is the thin line where several of these factors balance each other, as trust-building will result in a loyal customer base.
Future Trends in Data Analytics for Food Delivery Apps
The future of data analytics in apps will revolutionize the food delivery industry and is getting better with time. Some of the key trends going on right now include the integration of AI and ML into personalization. Data analytics study user data, such as order history and preferences, to allow your app to make recommendations to amaze your customer and increase his ordering frequency.
With real-time analytics, applications will have the ability to work around routing and time for an immense improvement in their operational efficiency. Predictive analytics also becomes crucial for predicting peaks in demand so that inventory and staffing can be done well in advance.
Another exciting use case is the sentiment analysis coming from social media and customer feedback. With insights into customers’ feelings and reactions, food delivery services refine their marketing strategy, focusing on improving user experience overall.
Finally, blockchain has emerged as a promising tool to be used for maintaining the traceability of food items with transparency, which is a yardstick for quality and safety. As users tend to grow more health-conscious, data analytics will continue to drive this sector into the future with marked transparency, personalization, and efficiency, building trust and loyalty among users.