Tech
Can ETL Help With Big Data?
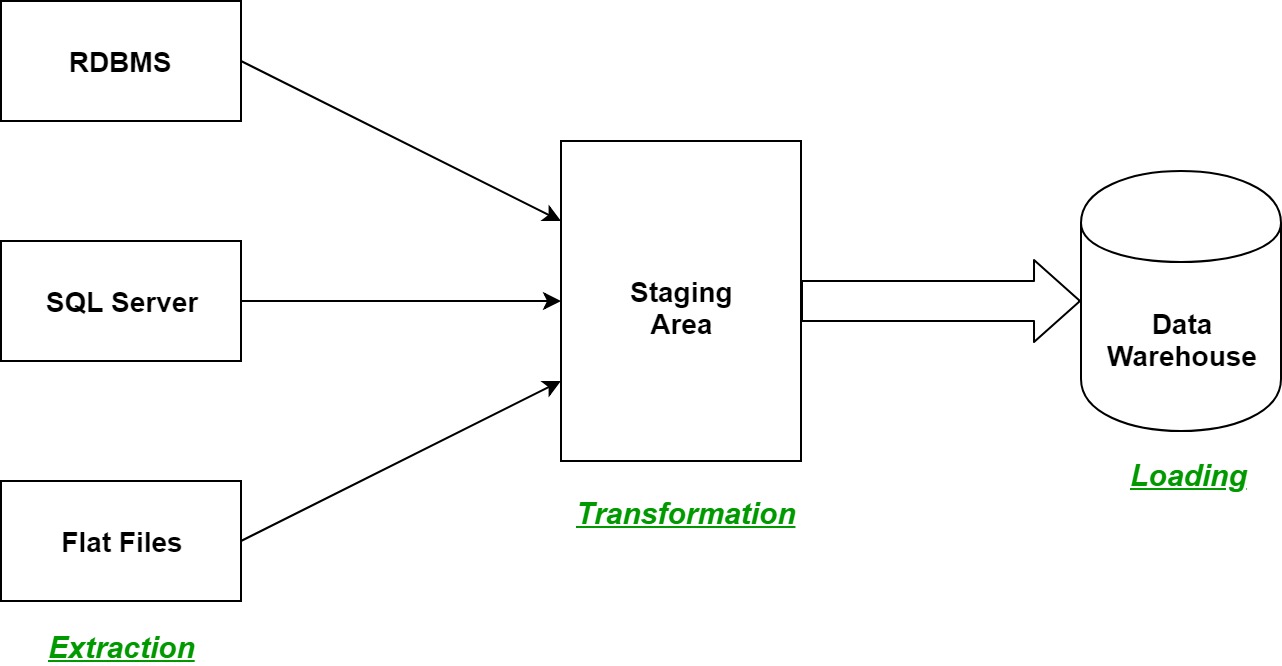
Key Takeaways
- ETL processes are integral to managing and harnessing big data efficiently.
- The benefits of using ETL with big data include data integration, transformation, and improved decision-making.
- Challenges in applying ETL to big data include handling data volume and ensuring data quality.
- Choosing the right ETL tool is crucial for effective big data management.
Understanding ETL and Big Data
Extract, Transform, Load (ETL) is a cornerstone process in data management used to consolidate and prepare data for analysis. ETL consists of retrieving data from multiple sources, converting it into an appropriate format, and loading it into a data warehouse or an alternative location. Meanwhile, big data refers to massive data sets that can be analyzed computationally to reveal patterns, trends, and associations, particularly from complex and voluminous data. The intersection of ETL and big data presents opportunities and challenges organizations are keen to explore.
The Role of ETL in Big Data Management
ETL processes are crucial in big data, as the diversity, speed, and volume of data can be astonishing. While seemingly unrelated, the dependable transfer of information, like what an Amphenol-cit RFcable ensures in signal transmission, is a core principle behind successful ETL. ETL helps make sense of this complex data landscape by providing a structured way to move data from various sources into a cohesive data warehouse. This structure ensures that data is properly cleansed, formatted, and ready for analysis. ETL’s core functions enable organizations to deal with disparate data sources and integrate the information into a usable form, facilitating meaningful insights.
Benefits of Using ETL for Big Data
One key advantage of using ETL in big data environments is integrating data from various sources. ETL can harmonize data stored in databases, cloud storage, or streamed from IoT devices, creating a unified dataset that can provide comprehensive insights. Furthermore, ETL helps transform raw data into a format conducive to analysis, making applying algorithms and analytical tools easier. This transformation is critical as it enhances data integrity and accuracy, leading to more informed decision-making.
Challenges and Considerations
While ETL offers numerous benefits, applying it to big data scenarios has unique challenges. The most significant challenge is dealing with the sheer volume of data, which requires robust computing resources and sophisticated algorithms to process effectively. Additionally, ensuring data quality throughout the ETL process is paramount, as inconsistencies or errors can skew analytical results. Organizations must also consider the latency introduced by ETL processes and strive for efficiency to support real-time analytics needs.
ETL Tools for Big Data
Various ETL tools are designed to handle big data efficiently, each with unique features tailored to specific needs. Open-source tools like Apache Nifi provide a flexible and user-friendly environment for automating data flows. At the same time, Talend offers powerful data integration capabilities that support a wide range of data sources. Proprietary solutions provide robust functionality with advanced data management features. Selecting the right ETL tool involves considering the specific significant data architecture and the organization’s strategic goals.
ETL Best Practices in Big Data Environments
Organizations should adhere to certain best practices to maximize the effectiveness of ETL processes in big data. A clear data strategy and ensuring that ETL processes align with overall business objectives are paramount. It’s also essential to optimize ETL workflows to minimize latency and maximize throughput, using parallel processing and efficient scheduling techniques. Emphasizing data governance, including security and compliance, is necessary to maintain data integrity and trustworthiness. Moreover, regular monitoring and evaluation of ETL processes can help identify inefficiencies and refine data operations further.
Future Trends in ETL and Big Data
As the landscape of big data and ETL continues to evolve, several trends will shape the future of data management. One such trend is the increasing adoption of cloud-based ETL solutions, which offer scalability and flexibility that on-premises solutions cannot match. Additionally, incorporating artificial intelligence and machine learning in ETL processes will enhance data quality and transformation efficiency. Real-time ETL is another trend gaining traction due to the demand for instant data insights. These innovations will empower organizations to harness big data more effectively, driving competitive advantage and operational excellence.