News
How to Leverage Data Analytics in Your Food Delivery App
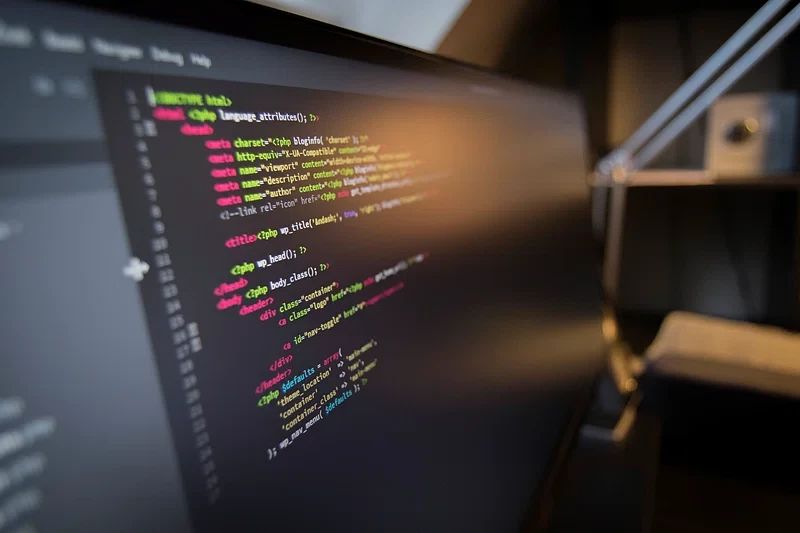
Introduction to Data Analytics in Food Delivery Apps
In today’s fast-paced digital landscape, food delivery apps are transforming how we enjoy our favorite meals. At the heart of this revolution lies data analytics — a powerful tool that enables businesses to better understand their customers, streamline operations, and enhance user experiences. By harnessing vast amounts of data generated through user interactions, food delivery apps can gain invaluable insights into customer preferences, order patterns, and market trends. For instance, utilizing services from https://celadonsoft.com/solutions/food-delivery-app-development-services can help in implementing advanced analytics features that drive customer satisfaction and loyalty.
Data analytics allows businesses to identify key performance indicators (KPIs) that drive success, such as the most popular dishes, peak order times, and customer demographics. This information helps app developers optimize their services, ensuring that users receive personalized recommendations and timely deliveries. Furthermore, predictive analytics can forecast demand, allowing restaurants and delivery services to manage inventory more efficiently and reduce food waste.
As competition intensifies in the food delivery sector, leveraging data analytics is not just a luxury — it’s a necessity. By embracing this technology, companies can foster loyalty, enhance user satisfaction, and ultimately drive growth in an increasingly crowded market. In the following sections, we will explore practical strategies for integrating data analytics into your food delivery app to maximize its potential.
Importance of Data Analytics for Food Delivery Services
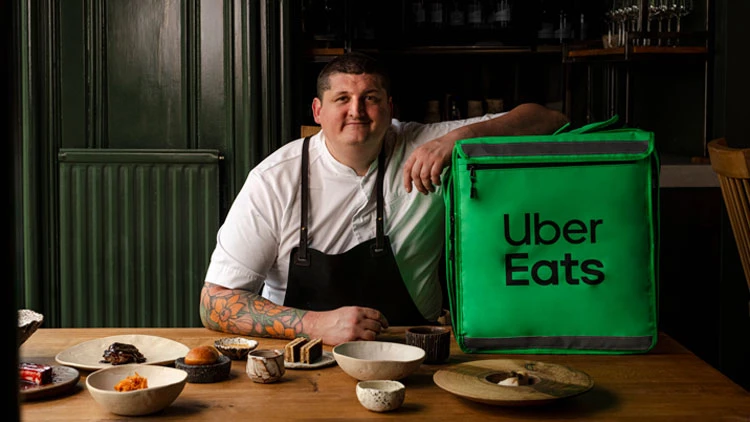
In the fast-paced world of food delivery services, data analytics has emerged as a game-changer, offering insights that can significantly enhance operational efficiency and customer satisfaction. The importance of data analytics lies in its ability to transform raw data into actionable strategies. By analyzing consumer behavior, delivery patterns, and peak ordering times, businesses can tailor their offerings to meet the specific needs of their customers.
For instance, data analytics allows food delivery apps to identify favorite dishes or cuisines in various demographics, enabling personalized marketing campaigns that resonate with users. Additionally, it helps optimize delivery routes, reducing wait times and improving service reliability, which is crucial in retaining customers in a competitive market.
Moreover, with real-time analytics, companies can track performance metrics and customer feedback, allowing for swift adjustments and improvements. Understanding trends and seasonality can also aid in inventory management, ensuring that popular items are always available while minimizing waste. Ultimately, leveraging data analytics not only enhances the customer experience but also drives profitability and fosters long-term growth in the food delivery sector.
Key Metrics to Track in Food Delivery Apps
- Order Conversion Rate: Measure the percentage of users who complete a purchase after adding items to their cart. A low rate may indicate issues with your checkout process or app usability.
- Customer Retention Rate: This metric reflects how many customers return to use your app again. High retention signifies customer satisfaction and loyalty, while low retention may point to areas needing improvement.
- Average Order Value (AOV): Calculate the average amount spent per order. Increasing AOV can significantly boost revenue, so consider strategies like upselling and cross-selling to enhance this figure.
- Delivery Time: Track the average time it takes for orders to be delivered. Timeliness is crucial in the food industry, and faster delivery can enhance customer satisfaction.
- Customer Satisfaction Score (CSAT): Collect feedback from users to understand their satisfaction levels. This score can provide insights into areas for improvement.
Types of Data to Collect from Users
To effectively leverage data analytics in your food delivery app, it’s crucial to identify and collect various types of user data that can enhance the customer experience and drive business growth.
First, gather demographic information such as age, gender, and location. This data helps tailor marketing strategies and menu offerings to meet the preferences of different customer segments.
Next, track user behavior within the app. Pay attention to order history, browsing patterns, and time spent on specific sections. This information reveals popular dishes, peak ordering times, and potential bottlenecks in the user journey.
Additionally, collect feedback through ratings and reviews. Understanding customer satisfaction levels and areas for improvement is vital for retaining users and attracting new ones.
Don’t overlook transaction data, including payment methods and average order values. Analyzing this can uncover trends that inform pricing strategies and promotions.
Lastly, consider integrating social media data to gauge customer sentiment and engagement. By combining these data types, your app can create a personalized experience that not only meets user needs but also enhances loyalty and increases revenue.
Utilizing Customer Feedback for Data-Driven Decisions
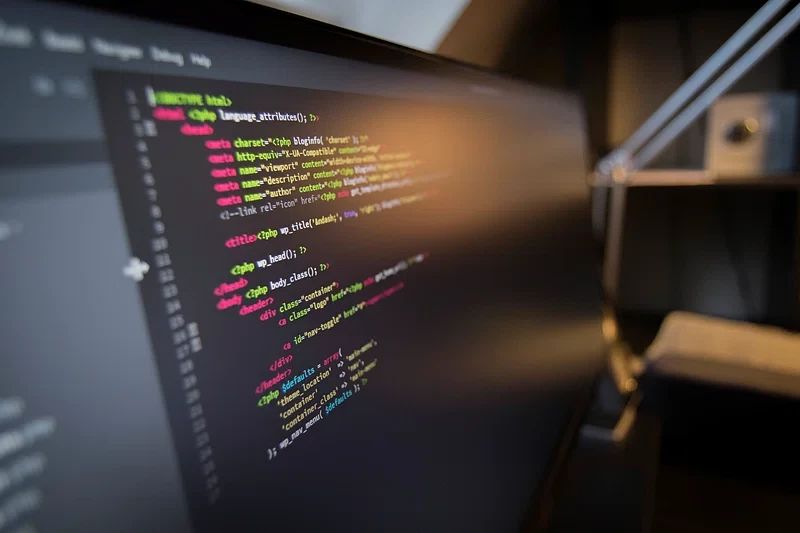
In the fast-paced world of food delivery, understanding customer sentiment is crucial for success. Leveraging customer feedback can transform raw data into actionable insights, driving your app’s growth and enhancing user experience. Start by integrating feedback mechanisms directly into your app — simple star ratings, detailed reviews, or even quick surveys after order completion can provide invaluable data.
Once you gather this feedback, employ analytics tools to categorize and analyze the responses. Look for common themes and trends, such as recurring complaints about delivery times or praise for specific dishes. This qualitative data can help identify areas needing improvement, such as optimizing delivery routes or enhancing menu offerings.
Moreover, consider implementing sentiment analysis algorithms to gauge customer emotions behind the feedback. This approach not only highlights satisfaction levels but also uncovers underlying issues that may not be immediately visible.
By continuously monitoring and responding to customer feedback, you create a dynamic feedback loop that fosters loyalty. Customers appreciate when their voices are heard, leading to higher retention rates and increased referrals. In a competitive landscape, those who listen closely and act decisively will rise above the rest.
Enhancing User Experience through Personalization
In the competitive landscape of food delivery apps, personalization has emerged as a key differentiator, and data analytics plays a crucial role in crafting tailored user experiences. By leveraging customer data — such as order history, preferences, and even time of day — apps can curate personalized menus that resonate with individual tastes. For instance, if a user frequently orders vegan dishes, the app can highlight similar options or recommend new vegan restaurants, creating a sense of familiarity and anticipation.
Moreover, predictive analytics can forecast cravings based on user behavior. If a customer tends to order pizza on Friday evenings, the app can send timely notifications or exclusive deals just as the weekend approaches, subtly nudging them towards a purchase.
Additionally, incorporating machine learning algorithms can enhance the personalization journey by continuously learning from user interactions, thereby evolving recommendations over time. By segmenting users into distinct categories — such as health-conscious eaters, adventurous foodies, or budget-conscious diners — apps can ensure that each user feels uniquely catered to, fostering loyalty and increasing engagement. Ultimately, when customers feel understood and valued, they are more likely to return, turning casual diners into devoted patrons.
Optimizing Delivery Operations with Data Insights
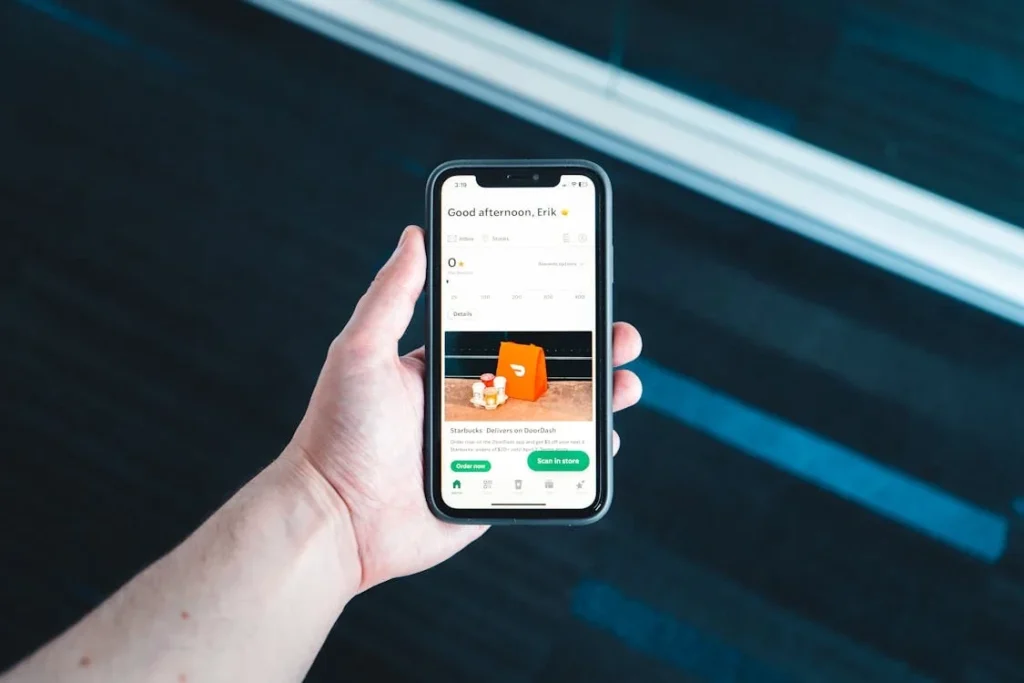
- Route Optimization: Utilize real-time traffic data and historical delivery patterns to identify the most efficient routes, reducing delivery times and fuel costs.
- Demand Forecasting: Analyze order trends and seasonal fluctuations to predict peak times, allowing for better staffing and inventory management.
- Performance Metrics: Track key performance indicators (KPIs) such as delivery time, order accuracy, and customer feedback to identify areas for improvement.
- Customer Segmentation: Use data to categorize customers based on preferences and behaviors, enabling personalized marketing and service offerings.
- Driver Performance Analysis: Monitor delivery personnel using data insights to ensure accountability and enhance training programs, ultimately improving service quality.
Case Studies: Successful Implementation of Data Analytics
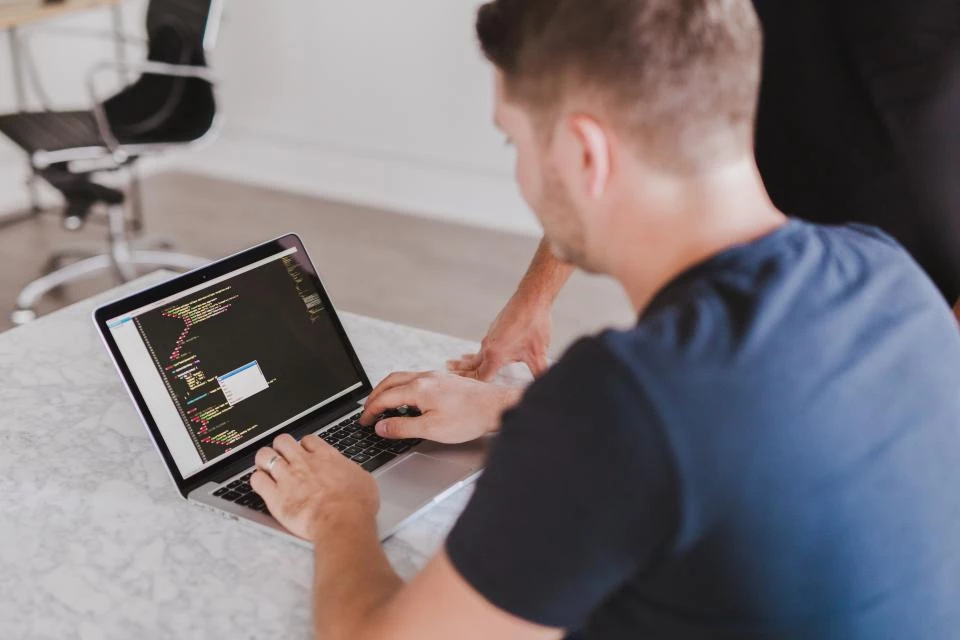
In the competitive landscape of food delivery apps, several companies have harnessed data analytics to enhance their offerings and drive growth. Take, for example, DoorDash. By leveraging customer data, the platform has fine-tuned its delivery routes, reducing delivery times by an impressive 30%. Their predictive analytics model anticipates peak times and customer preferences, ensuring that popular items are readily available, thus boosting order volume.
Another notable case is Uber Eats, which employs machine learning algorithms to analyze customer behavior and optimize menu recommendations. By examining past orders, user interactions, and even social media trends, the app curates personalized suggestions that not only improve user satisfaction but also increase average order sizes.
Meanwhile, Grubhub has utilized data analytics to identify and partner with local restaurants based on community preferences, creating a win-win situation for both consumers and businesses. This strategic approach not only enhances customer loyalty but also expands Grubhub’s market reach.
These case studies exemplify how thoughtful implementation of data analytics can lead to improved operational efficiency, heightened customer engagement, and ultimately, increased profitability in the food delivery sector.
Challenges in Leveraging Data Analytics
Leveraging data analytics in food delivery apps can be a game-changer, yet it comes with its own set of challenges. One significant hurdle is data quality. Inaccurate or incomplete data can skew results, leading to misguided business decisions. For instance, if user preferences are incorrectly logged, it could affect menu offerings and customer satisfaction.
Another challenge is the integration of disparate data sources. Food delivery apps often gather data from multiple channels, including customer feedback, order history, and social media. Ensuring that these various sources communicate effectively to provide a holistic view can be complex and resource-intensive.
Moreover, the fast-paced nature of the food delivery industry can overwhelm analytics systems. Real-time data processing is essential for understanding shifting consumer preferences and market trends. However, maintaining the infrastructure to support this can be costly and technically demanding.
Lastly, data privacy concerns loom large. With increasing regulations like GDPR, food delivery apps must navigate the fine line between leveraging data for personalized experiences and respecting user privacy. Balancing these factors is crucial for building trust and maintaining a loyal customer base.
Future Trends in Data Analytics for Food Delivery Apps
As the food delivery industry continues to evolve, the future of data analytics within apps is poised to bring transformative changes. One significant trend is the integration of artificial intelligence (AI) and machine learning (ML) to enhance personalization. By analyzing user data, such as order history and preferences, apps can tailor recommendations that not only delight customers but also increase order frequency.
Moreover, real-time data analytics will enable apps to optimize delivery routes and times, significantly improving operational efficiency. Predictive analytics will become crucial in anticipating demand spikes, allowing businesses to manage inventory and staffing proactively.
Another exciting development is the use of sentiment analysis derived from social media and customer feedback. By understanding customer emotions and reactions, delivery services can refine their marketing strategies and enhance the overall user experience.
Finally, blockchain technology is gaining traction for its ability to provide transparent tracking of food sources, ensuring quality and safety. As consumers become more health-conscious, data analytics will play a pivotal role in building trust and loyalty among users, driving the food delivery sector into a future defined by transparency, personalization, and efficiency.